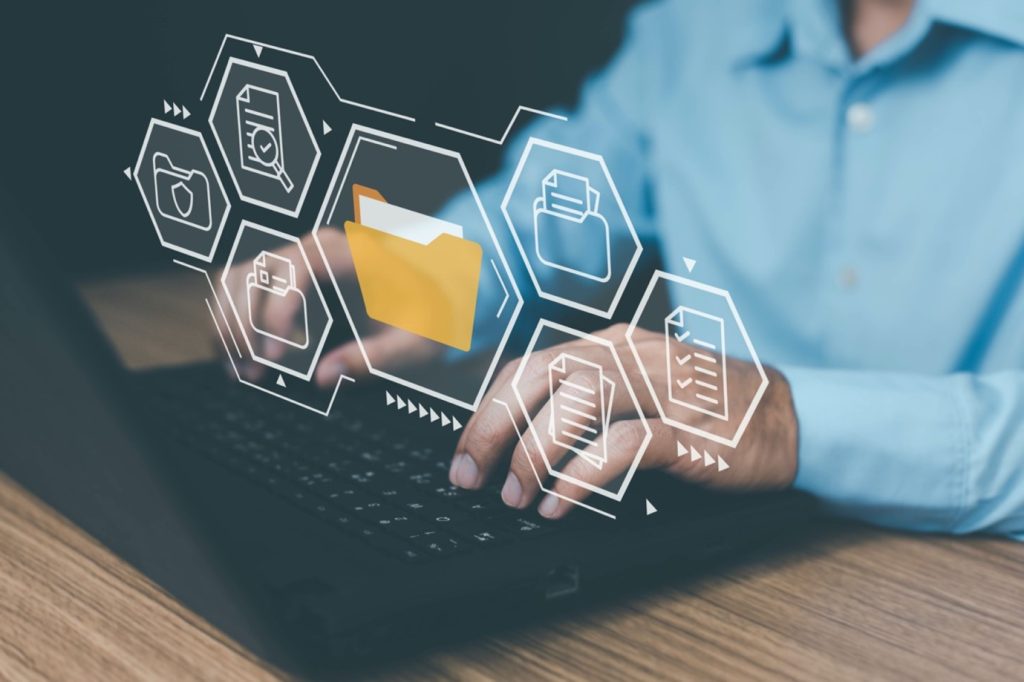
Introduction to Advanced Data Analytics
Every day 2.5 trillion bytes are generated worldwide and the amount of data is increasing rapidly [1]. What is spectacular about it is that about 90% of the data generated was only created in the last two years. It is forecast that the currently existing amount of data will even double every two years. It is not surprising that data is considered to be the most important raw material in the 21st century. Experts even say that data is the “new oil in the 21st century” [2,3].
In order to successfully position your company for the future and to implement digitization, data-driven business models and a digital mindset are required. Because only with the ability to derive intelligent decisions and actions from data will added value be generated for your company. This article compares business intelligence with advanced analytics. It shows what characterizes advanced analytics and how successful companies use advanced analytics in practice.
Every day 2.5 trillion bytes are generated worldwide and the amount of data is increasing rapidly [1]. What is spectacular about it is that about 90% of the data generated was only created in the last two years. It is forecast that the currently existing amount of data will even double every two years. It is not surprising that data is considered to be the most important raw material in the 21st century. Experts even say that data is the “new oil in the 21st century” [2,3].
Business Intelligence (BI) and Advanced Analytics
The term Business Intelligence (BI) bezeichnet refers to the examination and visualization of previous results [4]. The aim is to evaluate past events and to increase understanding of the interrelationships and essential influencing factors. This assumes that companies systematically collect, integrate, process, analyze and visualize their operational information [5]. A characteristic of BI is the use of key performance indicators (KPI), target / actual comparisons and scorecards. This approach is that Descriptive Analytics and has the lowest level of maturity in the analytics maturity curve (see Figure 1). The reason is that only the past is described and what has happened is examined [6]. For example, monthly sales figures achieved by a discounter serve as a basis for discussion for future decisions of the branch manager [7].
Descriptive analytics tools include, for example, relational databases, spreadsheets and operational data stores. A prominent tool is Microsoft Power BI. The tool supports users, cloud-based or locally (on-premise), in evaluating and visualizing data, such as creating management reports.

Figure 1: Differentiation between central analytics approaches in the maturity model (based on [6; 7; 8]
According to the maturity model, a clear distinction can be drawn between the classic, traditional approach of descriptive analytics and the more progressive advanced analytics. Advanced data analytics is divided into the two components predictive analytics and prescriptive analytics.
The terms are defined as follows:
- Predictive Analytics extracts information from data with the aim of predicting patterns and trends. It goes further than the classic business intelligence approach, as forecasts are modeled with regard to the future. So the horizon is directed towards future events. The relationships between parameters and target variables of past events are used to forecast unknown results [7]. In the maturity model (see Figure 1), predictive analytics is to be classified on a higher level than descriptive analytics due to its higher complexity and the potentially derivable business value. Let’s take the example of the discounter again: With predictive analytics, the added value is not in visualizing past sales. The differentiating feature is that expected sales figures are outlined with forecasts and future developments are modeled [7].
- The area of prescriptive analytics is at the highest level in the maturity model. Algorithms suggest concrete measures to achieve desired results in the future [7]. This means that the forecast from the area of predictive analytics is converted into specific recommendations for action. This could include recommending a marketing campaign to an executive at a specific time to maximize operational results.
In predictive and prescriptive analytics, the focus is shifting from evaluating past events to being more future-oriented. This enables companies to proactively control their business processes and influence future developments by choosing suitable measures [9].
Table 1 shows the most important methods and common tools of advanced analytics:
Table 1: Advanced AnalyticsTools for data analysis
Tools and analysis techniques of advanced analytics |
Deep Learning / Machine Learning (Supervised & Unsupervised Learning) |
(Convolutional) Neural Networks |
Text-Mining |
Data-Mining |
Clusterverfahren |
Decision trees |
How do successful companies use advanced analytics in practice?
Erfolgreiche Unternehmen wissen, dass sie mit ausgewählten Advanced Analytics Lösungen ihre Wettbewerbsfähigkeit in der heutigen durch zunehmende Digitalisierung geprägten Arbeitswelt steigern können [6]. Sie nutzen fortschrittlichste Werkzeuge, um schnellere und bessere Entscheidungen treffen zu können. Da diese auf Daten basieren, erhöht dies ebenfalls die Qualität der Entscheidung.
The following aspects are important to consider so that your company gets the maximum benefit from advanced analytics:
- Human:
Advanced analytics requires the collaboration of several experts from different specialist departments. The embedding of advanced analytics in the company creates new roles. Therefore, in addition to professional and technical skills, competencies with regard to communication and presentation are necessary. Teams are more interdisciplinary than ever. This means that data scientists, data engineers, statisticians, mathematicians and experts from the field of business knowledge work together to solve a problem.
This diversity is a great asset. However, it is crucial that the operational experts critically question the results and forecasts achieved and interpret them. Close ties and interdisciplinary exchange between advanced analytics experts and operational specialists deliver the best results.
The decision-makers in the company have a key task, because the implementation and anchoring of advanced analytics requires a corporate culture that is geared towards this. It is important that managers encourage their teams and developers to approach generated forecasts and recommendations in an unbiased and open manner. Business leaders have to create a high level of acceptance and tolerance for recommendations from algorithms. The commitment ensures that the trust of your employees in the new technological possibilities and data-based decisions grows.
- Processes:
The processes (from data acquisition to the creation of added value) in advanced analytics must be clearly structured and integrated into the organization [10]. It is crucial to be open to data and have the ability to collect it. An agile mindset and flexibility are required here. Rigid ways of thinking and stereotyped thinking is out of place.
The functionality of the approaches can be shown by means of proof of concepts. The knowledge gained from the evaluation enables continuous improvement to be driven forward.
- Modern technologies:
The last prerequisite for the success of advanced analytics is a modern infrastructure and working with modern technologies. Databases naturally play a central role against the background of the growing number of networked systems, devices and company information.
Data lakes are becoming increasingly important for the storage of a large volume of data, as they can provide unknown knowledge for several company departments and users [11]. In addition, powerful and reliable networks are required for data transmission.
In addition to suitable visualization tools (such as Microsoft Power BI), tools for creating algorithms are required. These include, for example, software and integrated development environments such as
- Azure Machine Learning Workbench,
- Amazon SageMaker,
- IBM Watson Studio,
- Google Cloud AI Platform,
- DataRobot, Python (Anaconda),
- R, RapidMiner,
- KNIME Analytics Platform, oder
- MATLAB.
The following table finally shows some exemplary use cases of predictive and prescriptive analytics in different departments.
Table 2: Overview of application examples of predictive analytics and prescriptive analytics
Department | Predictive Analytics | Prescriptive Analytics |
HR | Recruiting suitable new employees or predicting the likelihood of colleagues changing companies [12] | Increase in company loyalty: initiation of countermeasures to avoid employees who are at risk of switching [15] |
Finance | Fraud prevention: preventing vulnerability to fraudulent transactions | Automatic triggering of purchases or sales in the event of price fluctuations in algorithmic trading [16] |
Production / assembly | Predictive Maintenance: Predictive maintenance in the production of cylinder heads at Daimler [13] | Optimization of logistics and internal merchandise management [17] |
distribution | Forecast sales planning based on customer behavior models [14] | Optimiertes Bestandsmanagements [18] |
Conclusion
Advanced Analytics is the next evolutionary stage in Business Intelligence and enables future-oriented, proactive management of the company. With predictive and prescriptive analytics, better and better quality decisions can be made. So it is high time to deal intensively with these key technologies and the associated tools and to invest in these modern technologies.